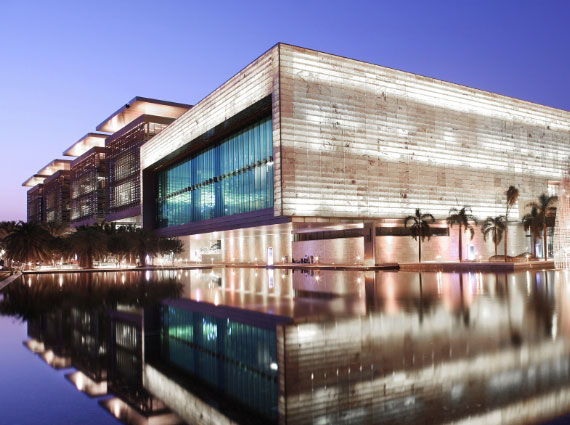
Project Details
Program
Computer Science
Field of Study
computer science, mathematics
Division
Computer, Electrical and Mathematical Sciences and Engineering
Faculty Lab Link
Project Description
Deep learning models provide state of the art performance on many practical machine learning tasks. However, there is a large gap between our theoretical / conceptual understanding and practice.
The intern will work in one of the follow areas, depending on interest and background:
- Deep learning models
- Adversarial attacks and robustness
- Optimization for deep learning
- Generalization of deep learning
- GANs
- Model compression
About the Researcher
Peter Richtarik
Professor, Computer Science
Affiliations
Education Profile
- PhD, Operations Research, Cornell University, 2007
- MS, Operations Research, Cornell University, 2006
- Mgr, Mathematics, Comenius University, 2001
- Bc, Management Comenius University, 2001
- Bc, Mathematics, Comenius University, 2000
Research Interests
Prof. Richtarik's research interests lie at the intersection of mathematics, computer science, machine learning, optimization, numerical linear algebra, high performance computing and applied probability. He is interested in developing zero, first, and second-order algorithms for convex and nonconvex optimization problems described by big data, with a particular focus on randomized, parallel and distributed methods. He is the co-inventor of federated learning, a Google platform for machine learning on mobile devices preserving privacy of users' data.Selected Publications
- R. M. Gower, D. Goldfarb and P. Richtarik. Stochastic block BFGS: squeezing more curvature out of data, Proceedings of The 33rd International Conference on Machine Learning, pp. 1869-1878, 2016
- J. Konecny, J. Liu, P. Richtarik and M. Takac. Mini-batch semi-stochastic gradient descent in the proximal setting, IEEE Journal of Selected Topics in Signal Processing 10(2), 242a-255, 2016
- P. Richtarik and M. Takac. Parallel coordinate descent methods for big data optimization Mathematical Programming 156(1):433a-484, 2016
- R. M. Gower and P. Richtarik. Randomized iterative methods for linear systems, SIAM Journal on Matrix Analysis and Applications 36(4):1660-1690, 2015
- O. Fercoq and P. Richtarik. Accelerated, parallel and proximal coordinate descent. SIAM Journal on Optimization 25(4):1997a-2023, 2015
Desired Project Deliverables
Ideally contribution to a research paper.
We are shaping the
World of Research
Be part of the journey with VSRP
3-6 months
Internship period
100+
Research Projects
3.5/4
Cumulative GPA
310
Interns a Year