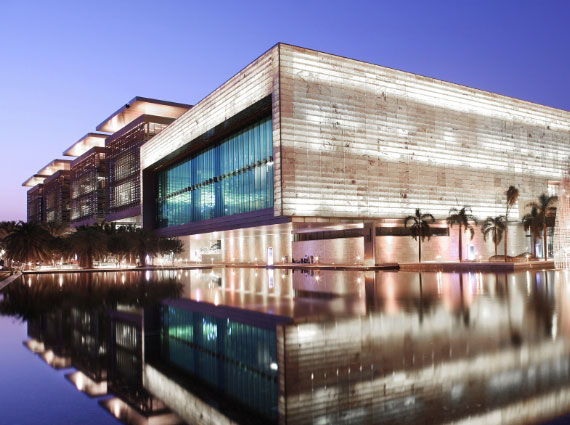
Project Details
Program
Applied Mathematics and Computer Science
Field of Study
Computational and Applied Mathematics
Division
Computer, Electrical and Mathematical Sciences and Engineering
Faculty Lab Link
Project Description
The student will work on machine learning techniques applied to the study of dynamical systems.
Specifically, the student will use different Neural Network architectures to approximate the equations governing a given system's evolution.
This evolution may be intrinsically random, or we may add randomness to contemplate the possibility of approximating a large dimensional system by a low dimensional one.
He will also test this methodology in several applications and report his results to get familiar with the problem and its input data.
About the Researcher
Raul Tempone
Professor, Applied Mathematics and Computational Science
Affiliations
Education Profile
- Ph.D. Numerical Analysis, Royal Institute of Technology, 2002
- M.S. Engineering Mathematics, Universidad de la Republica, Montevideo, Uruguay, 1999
- B.S. Industrial and Mechanical Engineering, Universidad de la Republica, Montevideo, Uruguay, 1995
Research Interests
Raul Tempone's research interests are in the mathematical foundation of computational science and engineering. More specifically, he has focused on a posteriori error approximation and related adaptive algorithms for numerical solutions of various differential equations, including ordinary differential equations, partial differential equations, and stochastic differential equations. He is also interested in the development and analysis of efficient numerical methods for optimal control, uncertainty quantification and bayesian model calibration, validation and optimal experimental design. The areas of application he considers include, among others, engineering, chemistry, biology, physics as well as social science and computational finance.Selected Publications
- Hoel, H., Shaimerdenova, G., & Tempone, R. (2022). Multi-index ensemble Kalman filtering. Journal of Computational Physics, 111561.
- Cramer, E., Mitsos, A., Tempone, R., & Dahmen, M. (2022). Principal component density estimation for scenario generation using normalizing flows. Data-Centric Engineering, 3.
- Kiessling, J., StrA¶m, E., & Tempone, R. (2021). Wind field reconstruction with adaptive random Fourier features. Proceedings of the Royal Society A: Mathematical, Physical and Engineering Sciences, 477(2255). doi:10.1098/rspa.2021.0236
- Espath, L., Kabanov, D., Kiessling, J., & Tempone, R. (2021). Statistical learning for fluid flows: Sparse Fourier divergence-free approximations. Physics of Fluids, 33(9), 097108. doi:10.1063/5.0064862
- Nadhir Ben Rached, Abla Kammoun, Mohamed-Slim Alouini, Raul Tempone , On the Efficient Simulation of Outage Probability in a Log-normal Fading Environment,IEEE Transactions on Communications 65 Issue: 6, 2017
- F. Ruggeri, Z. Sawlan, M. Scavino, R. Tempone, A hierarchical Bayesian setting for an inverse problem in linear parabolic PDEs with noisy boundary conditions, Bayesian Analysis, Advance Publication, 12 May 2016. doi: 10.1214/16-BA1007
- C. Bayer, J Happola, R. Tempone, Implied Stopping Rules for American Basket Options from Markovian Projection, arXiv:1705.00558v1, May 2017
- A. Haji-Ali, R. Tempone, ""Multilevel and Multi-index Monte Carlo methods for the McKean-Vlasov equation"", has been accepted for publication in Statistics and Computing. 20
- M. Iglesias, Z. Sawlan, M. Scavino, R. Tempone, C. Wood, Bayesian inferences of the thermal properties of a wall using temperature and heat flux measurements, accepted for publication in International Journal of Heat and Mass Transfer, Sep. 2017
Desired Project Deliverables
As the main project deliverable, we expect a scientific report describing the methodology developed in the internship and its numerical use in various applications. The working environment the student will use should include a GIT repository for all project-related materials to facilitate proper verification and feedback processes. These materials include, among others, the codes and the saved input-outputs corresponding to all tested cases
Recommended Student Background
Education in applied & computational mathematics
Education and possibly experience in machine learning and/or stochastic numerics
Experience with code development and software engineering skills, such as C++ and/or python
We are shaping the
World of Research
Be part of the journey with VSRP
3-6 months
Internship period
100+
Research Projects
3.5/4
Cumulative GPA
310
Interns a Year