Integration of reservoir simulation with deep learning for subsurface reservoir management
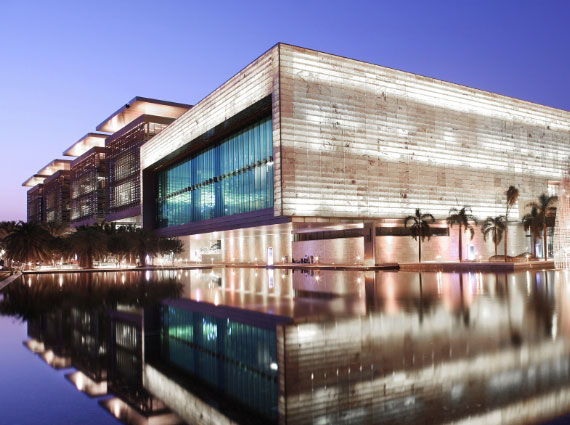
Project Details
Program
Energy Resources and Petroleum Engineering
Field of Study
Petroleum Engineering, Computational Mathematics, Machine Learning
Division
Physical Sciences and Engineering
Faculty Lab Link
Center Affiliation
Ali I. Al-Naimi Petroleum Engineering Research Center
Project Description
DSFT is a research team with diverse expertise including numerical modeling, machine/deep learning and energy system management. We are dedicating to technology development of advanced physics-driven numerical simulation and data-driven modeling for fluid flow in porous media. The goal of this visiting student project is to develop reservoir models to simulate the process of geological carbon storage, geothermal recovery or hydrogen storage, and ultimately use deep learning (e.g., convolutional/recurrent/graphic neural networks) or physics-informed neural networks to establish surrogate models for fast prediction of these nonlinear processes, and ultimately be ready for application of uncertainty quantification and also optimization.
We seek for self-motivated, dedicated and creative students who wants to address challenging energy and environmental related engineering problems, whose majors are from petroleum engineering, computational mathematics, machine learning or closely related fields. Desired qualification will be competitive students with good skills of reservoir simulation, python or Julia programming and deep learning.
About the Researcher
Bicheng Yan
Assistant Professor, Energy Resources and Petroleum Engineering
Affiliations
Education Profile
- Postdoctoral Research Associate, Los Alamos National Lab, 2020-2021
- Ph.D. Petroleum Engineering, Texas A&M University, 2017
- M.S. Petroleum Engineering, Texas A&M University, 2013
- B.S. Petroleum Engineering, China University of Petroleum (Beijing), 2009
Research Interests
Professor Yan's current research interests are physics-driven modeling and data-driven modeling for complex subsurface processes related to energy and environmental domains. He has worked in areas including reservoir simulation, multi-porosity modeling for flow and transport in heterogeneous porous media (mainly shale), integrating physics with data analytics in unconventional field and carbon storage management, history matching and stochastic optimization.Selected Publications
- Yan, B., Mi, L., Chai, Z., Wang, Y. et al. 2018. An Enhanced Discrete Fracture Network Model for Multiphase Flow in Fractured Reservoirs. Journal of Petroleum Science and Engineering, Vol 161: 667-682.
- Yan, B., Mi, L., Wang, Y., Tang, H. etl al. 2018. Multi-Porosity Multi-Physics Compositional Simulation for Gas Storage and Transport in Highly Heterogeneous Shales. Journal of Petroleum Science and Engineering, Vol 160: 498-509.
- Yan, B., Wang, Y., Killough, J. 2017. A Fully Compositional Model Considering the Effect of Nanopores in Tight Oil Reservoirs. Journal of Petroleum Science and Engineering, Vol 152: 675-682.
- Yan, B., Alfi, M., An, C., Cao, Y., Wang, Y., Killough, J.E. 2016. General Multi-Porosity Simulation for Fractured Reservoir Modeling. Journal of Natural Gas Science and Engineering, Vol 33: 777-791.
- Yan, B., Wang, Y., and Killough, J.E. 2015. Beyond Dual-Porosity Modeling for the Simulation of Complex Flow Mechanisms in Shale Reservoirs. Computational Geosciences, Vol 20 (1): 69-91.
Desired Project Deliverables
- report - prototype
Recommended Student Background
Reservoir simulation
Deep learning
Python programming
Flow in porous media
We are shaping the
World of Research
Be part of the journey with VSRP
3-6 months
Internship period
100+
Research Projects
3.5/4
Cumulative GPA
310
Interns a Year