Fine-Tuning of Foundation Models via Low-Rank Adaptation and Beyond
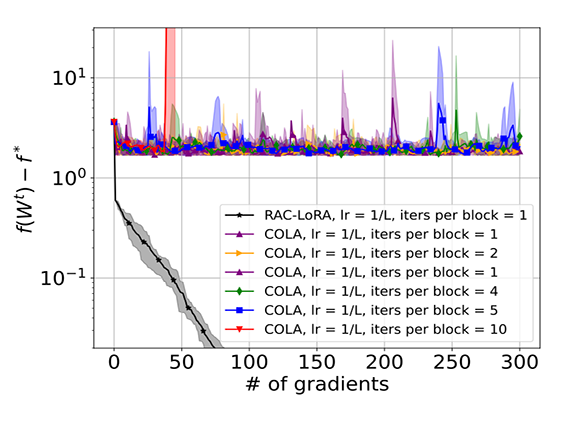
Project Details
Project Description
Fine-tuning has become a popular approach to adapting large foundational models to specific tasks. As the size of models and datasets grows, parameter-efficient fine-tuning techniques are increasingly important. One of the most widely used methods is Low-Rank Adaptation (LoRA), with adaptation update expressed as the product of two low-rank matrices. While LoRA was shown to possess strong performance in fine-tuning, it often under-performs when compared to full-parameter fine-tuning (FPFT). Although many variants of LoRA have been extensively studied empirically, their theoretical optimization analysis is heavily under-explored.
Edward J. Hu, Yelong Shen, Phillip Wallis, Zeyuan Allen-Zhu, Yuanzhi Li, Shean Wang, Lu Wang, Weizhu Chen. LoRA: Low-Rank Adaptation of Large Language Models, https://arxiv.org/abs/2106.09685, 2021
Tim Dettmers, Artidoro Pagnoni, Ari Holtzman, Luke Zettlemoyer. QLoRA: Efficient Finetuning of Quantized LLMs, https://arxiv.org/abs/2305.14314, 2023
Grigory Malinovsky, Umberto Michieli, Hasan Abed Al Kader Hammoud, Taha Ceritli, Hayder Elesedy, Mete Ozay, Peter Richtárik. Randomized Asymmetric Chain of LoRA: The First Meaningful Theoretical Framework for Low-Rank Adaptation, https://arxiv.org/abs/2410.08305, 2024
About the Researcher
Affiliations
Education Profile
- PhD, Operations Research, Cornell University, 2007
- MS, Operations Research, Cornell University, 2006
- Mgr, Mathematics, Comenius University, 2001
- Bc, Management Comenius University, 2001
- Bc, Mathematics, Comenius University, 2000
Research Interests
Prof. Richtarik's research interests lie at the intersection of mathematics, computer science, machine learning, optimization, numerical linear algebra, high performance computing and applied probability. He is interested in developing zero, first, and second-order algorithms for convex and nonconvex optimization problems described by big data, with a particular focus on randomized, parallel and distributed methods. He is the co-inventor of federated learning, a Google platform for machine learning on mobile devices preserving privacy of users' data.Selected Publications
- R. M. Gower, D. Goldfarb and P. Richtarik. Stochastic block BFGS: squeezing more curvature out of data, Proceedings of The 33rd International Conference on Machine Learning, pp. 1869-1878, 2016
- J. Konecny, J. Liu, P. Richtarik and M. Takac. Mini-batch semi-stochastic gradient descent in the proximal setting, IEEE Journal of Selected Topics in Signal Processing 10(2), 242a-255, 2016
- P. Richtarik and M. Takac. Parallel coordinate descent methods for big data optimization Mathematical Programming 156(1):433a-484, 2016
- R. M. Gower and P. Richtarik. Randomized iterative methods for linear systems, SIAM Journal on Matrix Analysis and Applications 36(4):1660-1690, 2015
- O. Fercoq and P. Richtarik. Accelerated, parallel and proximal coordinate descent. SIAM Journal on Optimization 25(4):1997a-2023, 2015
Desired Project Deliverables
Recommended Student Background
We are shaping the
World of Research
Be part of the journey with VSRP