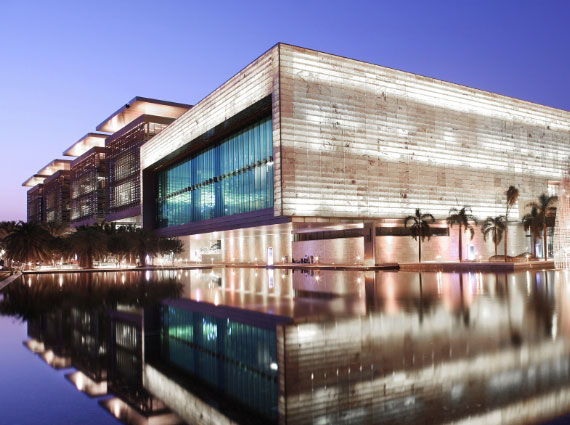
Project Details
Program
Computer Science
Field of Study
Computer Science, Mathematics or a related discipline
Division
Computer, Electrical and Mathematical Sciences and Engineering
Faculty Lab Link
Project Description
One of the most used algorithm to train deep neural networks is Adam. However, despite its empirical success, it is a poorly understood algorithm. In particular, existing mathematical theories fail to capture a quantifiable advantage over the classic stochastic gradient descent.
In this project, we will take a different route: Instead of studying Adam as a black-box under simplified assumptions, we will carefully analyze its empirical training dynamics, in particular in the first iterations. We aim at pinpointing the key differences between the training dynamics of Adam and the ones of stochastic gradient descent with momentum.
Later, using the gathered knowledge, we will formulate a mathematical model of its behavior.
About the Researcher
Francesco Orabona
Associate Professor, Computer Science
Affiliations
Education Profile
- Postdoctoral Researcher, University of Milano, 2010-2011
- Postdoctoral Researcher, IDIAP Research Institute, 2007-2009
- Ph.D., University of Genoa, 2007
- B.Sc. and M.Sc. (""Laurea""), University of Napoli ""Federico II"", 2003
Research Interests
Professor Orabona's research lies at the intersection of practical and theoretical machine learning. His research interests encompass online learning, optimization, and statistical learning theory. His current research focus is on designing ""parameter-free"" machine learning algorithms, which are algorithms that operate effectively without requiring costly parameter tuning.Selected Publications
- A. Cutkosky, H. Mehta, F. Orabona. a€œOptimal Stochastic Non-smooth Non-convex Optimization through Online-to-Non-convex Conversiona€. in: Proc. of the International Conference on Machine Learning. 2023
- N. Cesa-Bianchi and F. Orabona. a€œOnline Learning Algorithmsa€. In: Annual Review of Statistics and Its Application 8 (2021)
- A. Cutkosky and F. Orabona. a€œMomentum-Based Variance Reduction in Non-Convex SGDa€. in: Advances in Neural Information Processing Systems 32. 2019
- X. Li and F. Orabona. a€œOn the Convergence of Stochastic Gradient Descent with Adaptive Stepsizesa€. In: Proc. of the International Conference on Artificial Intelligence and Statistics (AISTATS). 2019
- F. Orabona and D. PA¡l. a€œCoin Betting and Parameter-Free Online Learninga€. In: Advances in Neural Information Processing Systems 29. 2016
Desired Project Deliverables
Original research – contribution to a research paper