Predicting Bacterial Antimicrobial Resistance Phenotypes from Genomic Biomarkers
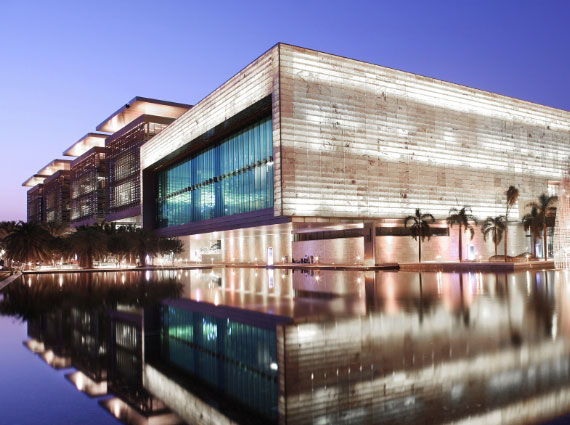
Project Details
Program
BioScience
Field of Study
Microbial genomics
Division
Biological and Environmental Sciences and Engineering
Faculty Lab Link
Center Affiliation
Computational Bioscience Research Center
Project Description
The Infectious Disease Epidemiology lab of Prof. Danesh Moradigaravand
is looking for student interns to work on a range of projects on the
intersection of machine learning and microbial genomics. We are interested
in understanding the evolution and epidemiology of antimicrobial resistance
strains recovered from natural sources, including clinical and environmental
sites. Antimicrobial resistance is a global health threat, expected to become
a leading cause of deaths worldwide within the next three decades. This is
predominately due to the rapid emergence of novel genetic variants, which
lead to new resistance mechanisms. The understanding of the genetic
repertoire of resistance is henceforth important to design new antimicrobial
strategies and develop new compounds. In this project, the student will
develop a machine learning-based predictive model to systematically
identify resistance determinants in bacterial genomes. The projects
involves integration of genomic and phenomic data, generated by high
throughput phenotypic assays using an off-the-shelf machine learning
method. The student will implement an entire predictive pipeline and deploy
the model as a data science solution. The project helps student gain
hands-on experience of programming, next generation sequencing data
analysis and machine learning
About the Researcher
Danesh Moradigaravand
Assistant Professor, Bioscience
Affiliations
Education Profile
- Research Associate, Wellcome Sanger Institute, Hinxton 2018
- PhD in Theoretical and Computational Biology, ETH Zurich 2014
- MSc in Molecular Biology, International Max Planck Research School Goettingen 2011
Research Interests
a€‹Professor Moradigaravand's research is on the evolution and epidemiology of infectious diseases, especially in the context of antimicrobial resistance. He utilises a broad range of genomic, phenomic and machine learning approaches to understand the dissemination of pathogens within and between environmental and clinical settings and to pinpoint genetic factors driving the evolution of pathogens. Moreover, he employs machine learning approaches for predicting bacterial complex phenotypic features, e.g. bacterial growth, antimicrobial resistance, and horizontal gene transfer, from genomic variantsSelected Publications
- Danesh Moradigaravand, Sandra Reuter, Veronique Martin, Sharon J Peacock, Julian Parkhill, The dissemination of multidrug-resistant Enterobacter cloacae throughout the UK and Ireland. Nat Microbiol, 2016. 1: p. 16173.
- Danesh Moradigaravand, Martin Palm, Anne Farewell, Ville Mustonen, Jonas Warringer, Leopold Parts, Prediction of antibiotic resistance in Escherichia coli from large-scale pan-genome data. PLoS Comput Biol, 2018. 14(12): p. e1006258
- Danesh Moradigaravand, Veronique Martin, Sharon J Peacock, Julian Parkhill, Evolution and Epidemiology of Multidrug-Resistant Klebsiella pneumoniae in the United Kingdom and Ireland. mBio, 2017. 8(1)
- Danesh Moradigaravand, Christine J Boinett, Veronique Martin, Sharon J Peacock, Julian Parkhill, Recent independent emergence of multiple multidrug-resistant Serratia marcescens clones within the United Kingdom and Ireland. Genome Res, 2016. 26(8): p. 1101-9.
- Sam Benkwitz-Bedford, Martin Palm, Talip Yasir Demirtas, Ville Mustonen, Anne Farewell, Jonas Warringer, Leopold Parts, Danesh Moradigaravand, Machine Learning Prediction of Resistance to Subinhibitory Antimicrobial Concentrations from Escherichia coli Genomes. mSystems, 2021. 6(4): p. e0034621.
Desired Project Deliverables
Develop and deploy a machine learning pipeline
Disclose a database of predictive biomarkers for antimicrobial resistance
Contribute to relevant publications
Recommended Student Background
Prior hands-on experience of writing high quality codes in R and Python
Familiarity with bash programing and running computational tasks on clouds
Familiarity with next generation sequencing technologies
Excellent problem-solving skills