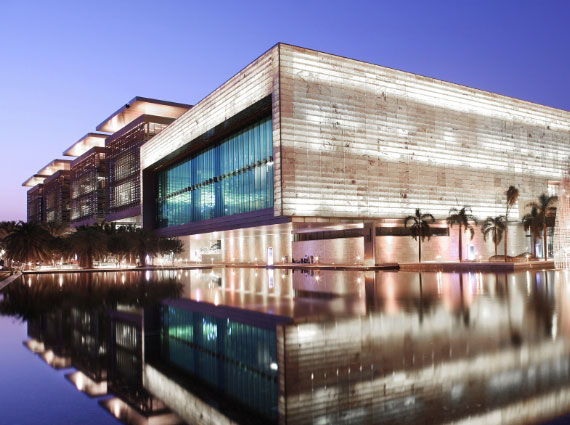
Project Details
Program
Computer Science
Field of Study
Computer Vision and Machine Learning
Division
Computer, Electrical and Mathematical Sciences and Engineering
Faculty Lab Link
Center Affiliation
Visual Computing Center
Project Description
Imagination has been the source of novel ideas that enable humanity to progress at an ever-faster rate. It is also is one of the key properties of human intelligence that enables us to generate creative products like music and art. Imagination is not only helpful for creating Art and Fashion, it also helps us see the world. The goal of this project is to focus on developing techniques that empower AI machines to see the world (computer vision)orto create novel products (e.g., fashion and art), hence the name Imagination Inspired Vision.Fore more context; see my talk on this topic, recorded at the University of Southern California; https://bluejeans.com/playback/s/jC9ksXIMhbHTw25sLghFgeGoWGNHcM 9VYc6QJQ8Z0yEq9X5aogZUWJ0AwDKz8hSR Vision-CAIR group at KAUST stands for Computer Vision- "C" Artificial Intelligence Research. "C" is for Content or Creative since we cover in our lab both Vision Content AI research and Vision- Creative AI research. For more information, please visit:http://www.mohamed-elhoseiny.com/vision-cair-group
About the Researcher
Mohamed Elhoseiny
Associate Professor, Computer Science
Affiliations
Education Profile
- Postdoctoral Researcher, Facebook AI Research (FAIR), 2017
- Ph.D., Rutgers University, 2016
- M.S., Rutgers University, 2014
- M.S., Ain Shams University, 2010
- B.E., Ain Shams University, 2006
Research Interests
Dr. Mohamed Elhoseiny is an Assistant Professor of Computer Science at the Visual Computing Center at KAUST (King Abdullah University of Science and Technology). Dr. Elhoseiny has collaborated with several researchers at Facebook AI Research including Marcus Rohrbach, Yann LeCun, Devi Parikh, Dhruv Batra, Manohar Paluri, Marc'Aurelio Ranzato, and Camille Couprie. He has also fruitfully teamed up with academic institutions including KULeuven (with Rahaf Aljundi and Tinne Tuytelaars), UC Berkeley (with Sayna Ebrahimi and Trevor Darrell), the University of Oxford (with Arslan Chaudry and Philip Torr), and the Technical University of Munich (with Shadi AlBarqouni and Nassir Navab). His primary research interests are in computer vision, the intersection between natural language and vision and computational creativity. Dr. Elhoseiny received his Ph.D. degree from Rutgers University, New Brunswick, in October 2016 under Prof. Ahmed Elgammal. His work has been widely recognized. In 2018, he received the best paper award for his work on creative fashion generation at ECCV workshop from Tamara Berg of UNC chapel hill and sponsored by IBM Research and JD AI Research. The work got also featured at the New Scientist Magazine and he co-presented it the Facebook F8 annual conference with Camille Couprie. His earlier work on creative art generation was featured by the New Scientist magazine and MIT technology review in 2017, HBO Silicon Valley TV Series ( season 5 episode 3) in 2018. His Creative AI artwork was featured/presented at the best of AI meeting 2017 at Disney (6000+ audience), Facebook's booth at NeurIPS 2017, and the official FAIR video in June 2018. His work on life-long learning was covered at the MIT technology review in 2018. In Nov 2018 and based on his 5-year work on zero-shot learning, Dr. Elhoseiny made significant participation in the United Nations Biodiversity conference (~10,000 audience from >192 countries and tens of important organization) on how AI may benefit biodiversity which reflects in both disease management and climate change. Dr. Elhoseiny received the Doctoral Consortium award at CVPR 2016 and an NSF Fellowship for his Write-a-Classifier project in 2014.Selected Publications
- Ji Zhang,Yannis Khaladis, Marcus Rohbrach, Manohar Paluri, Ahmed Elgammal, Mohamed Elhoseiny, a€œLarge-Scale Visual Relationship Understandinga€, AAAI, 2019
- Ramprasaath Selvaraju, Prithvijit Chattopadhyay, Mohamed Elhoseiny, Tilak Sharma, Dhruv Batra, Devi Parikh, Stefan Lee, a€œChoose your Neuron: Incorporating Domain Knowledge through Neuron Importancea€, ECCV, 2018
- Rahaf Aljundi, Francesca Babiloni, Mohamed Elhoseiny, Marcus Rohrbach, Tinne Tuytelaars, a€œMemory Aware Synapses: Learning what (not) to forgeta€, ECCV, 2018
- Yizhe Zhu, Mohamed Elhoseiny, Bingchen Liu, Ahmed Elgammal, a€œImagine it for me: Generative Adversarial Approach for Zero-Shot Learning from Noisy Textsa€, CVPR, 2018
- Ahmed Elgammal, Bingchen Liu, and Diana Kim, and Mohamed Elhoseiny, and Marian Mazzone, a€œThe Shape of Art History in the Eyes of the Machinea€, AAAI, 2018 (oral)
- Mohamed Elhoseiny, Francesca Babiloni, Rahaf Aljundi, Marcus Rohrbach, Tinne Tuytelaars, a€œExploring the Challenges towards Lifelong Fact Learninga€, ACCV, 2018
- Mohamed Elhoseiny*, Yizhe Zhu*, Han Zhang, Ahmed Elgammal, a€œLink the head to the ""peak'': Zero Shot Learning from Noisy Text descriptions at Part Precisiona€, International Conference on Computer Vision and Pattern Recognition, CVPR, 2017
- Ji Zhang*, Mohamed Elhoseiny*, Walter Chang, Scott Cohen, Ahmed Elgammal, ""Relationship Proposal Networks"", International Conference on Computer Vision and Pattern Recognition (CVPR), 2017, * equal contribution
- Mohamed Elhoseiny, Scott Cohen, Walter Chang, Brian Price, Ahmed Elgammal, a€œSherlock: Scalable Fact Learning in Imagesa€, AAAI Conference on Artificial Intelligence, 2017, acceptance rate 24%.
- Ahmed Elgammal and Bingchen Liu, Mohamed Elhoseiny, Marian Mazzone, a€œCreative Adversarial Networks: Generating ""Art"" by Learning About Styles and Deviating from Style Normsa€, International Conference on Computational Creativity, ICCC, 2017
Desired Project Deliverables
Develop imagination inspired solutions machine learning research for helping understanding and the creation of the unseen.
1) students should learn about machine learning, deep learning, andtherespective target application chosen for the internship.
2) students are expected to show capability to go from an idea to a working prototype; pushing the limits of what the state of the art can do.