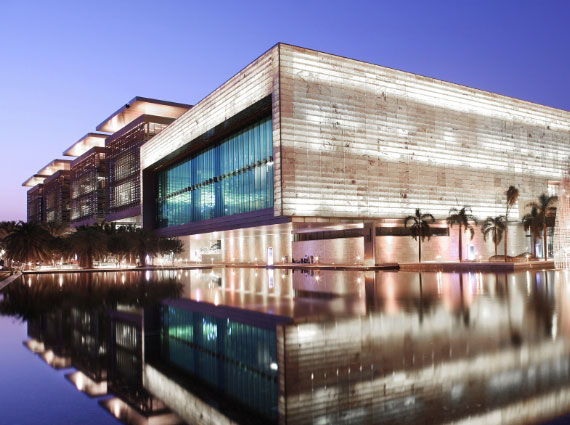
Project Details
Program
Computer Science
Field of Study
Computer Science
Division
Computer, Electrical and Mathematical Sciences and Engineering
Faculty Lab Link
Center Affiliation
Resilient Computing and Cybersecurity Center
Project Description
Federated learning (FL) is a novel paradigm enabling distributed machine learning (ML) model training, while ensuring that training data remains on individual clients. The increasing need for privacy makes FL a highly promising method spearheading the future of ML. Although theoretically elegant, FL faces significant hurdles when it comes to real-world implementation. The key obstacle towards wider proliferation of FL is an inherent heterogeneity of real-world settings. Clients that may participate in FL range from computationally limited Internet of Things (IoT) devices, over low-end smartphones, to high-end multicore GPU-powered smartphones and beyond. Furthermore, devices may host a range of different applications that are simultaneously competing for hosts’ limited resources. In this work we will for the first time quantify the effects of heterogeneity on FL performance in a real-world testbed. We will first conduct a survey of edge devices that are suitable for FL and assemble a representative, yet diverse, testbed at our KAUST premises. Building upon the existing open-source solutions, we will then program a FL training framework enabling rapid prototyping, experimentation, and measurements within our testbed. We will then conduct a set of experiments geared towards profiling the effect of device hardware, software, and usage heterogeneity of FL training accuracy, convergence time, fairness, and energy use. Finally, we will analyze the results and extract guidelines for efficient FL in heterogeneous environments.
About the Researcher
Marco Canini
Associate Professor, Computer Science
Affiliations
Education Profile
- Postdoctoral Researcher, EPFL, 2009-2012
- Ph.D., University of Genoa, 2009
- M.Sc. and B.Sc., University of Genoa, 2005
Research Interests
a€‹Professor Caninia€˜s research interests are in the principled construction and operation of large scale networked computer systems, specifically in distributed systems, large-scale computing and computer networking with emphasis on cloud computing and programmable networks. His current work focuses on improving networked systems design, implementation and operation along several vital properties such as reliability, performance, security and energy efficiency.Selected Publications
- Gupta, A. and MacDavid, R.and Birkner, R. and Canini, M. and Feamster, N. and Rexford, J. and Vanbever, L., 2016, An Industrial-Scale Software Defined Internet Exchange Point, Proceedings of 13th USENIX Symposium on Networked Systems Design and Implementation (NSDI '16), Community Award.
- Suresh, L. and Canini, M. and Schmid, S. and Feldmann, A., 2015, C3: Cutting Tail Latency in Cloud Data Stores via Adaptive Replica Selection, Proceedings of 12th USENIX Symposium on Networked Systems Design and Implementation (NSDI '15).
- Canini, M. and Venzano, D. and PereA¡Ani, P. and KostiA‡, D. and Rexford, J., 2012, A NICE Way to Test OpenFlow Applications, Proceedings of 9th USENIX Symposium on Networked Systems Design and Implementation (NSDI '12).
- Goma, E. and Canini, M. and Lopez Toledo, A. and Laoutaris, N. and KostiA‡, D. and Rodriguez, P. and StanojeviA‡, R. and YagA¼e ValentAn, P., 2011, Insomnia in the Access or How to Curb Access Network Related Energy Consumption, Proceedings of ACM SIGCOMM '11.
- Li, W. and Canini, M. and Moore, A. W. and Bolla, R., 2009, Efficient application identification and the temporal and spatial stability of classification schema, Computer Networks, 53(6), 790a-809, doi:10.1016/j.comnet.2008.11.016.
Desired Project Deliverables
- Physical testbed comprising a range of IoT and mobile devices.
- Programming framework for FL experimentation and evaluation.
- Executable scenarios encompassing different use-cases and levels of the underlying system heterogeneity.
- Report on the experimental results with metrics designed to profile the effect of system heterogeneity on holistic performance of FL.
- Guidelines for advancing FL in heterogeneous environments.