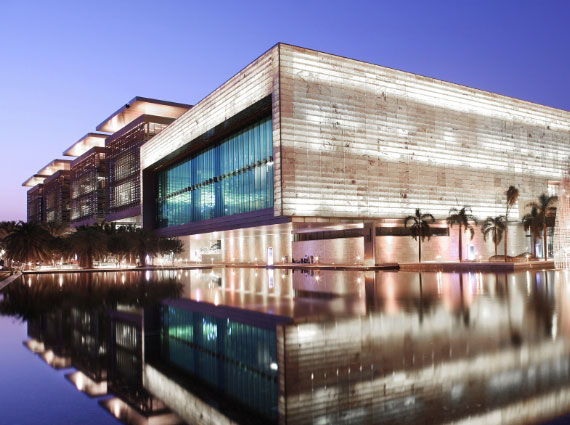
Project Details
Program
Computer Science
Field of Study
Computer Vision; Machine Learning
Division
Computer, Electrical and Mathematical Sciences and Engineering
Faculty Lab Link
Center Affiliation
Visual Computing Center
Project Description
Have you ever imagined having a robot that cooks every meal for you? Have you ever dreamt about experiencing a different world in the Metaverse? Have you ever expected to have your glasses tell you where you left your keys and how to navigate to your favorite restaurant step by step? If yes, egocentric video understanding is what can lead you there.
Egocentric videos are videos recorded from the first-person point of view, with the camera mounted on the head (e.g., GoPro) or smart glasses worn alongside the eyes (e.g., Google glasses), and they are what you actually see in your eyes. We need the AI system to automatically analyze and understand this type of videos to achieve the goals mentioned above. There are two key aspects in this problem: 1) large egocentric video data to fuel AI solutions; 2) effective techniques to generate correct predictions.
For the first aspect, our IVUL group has devoted two years’ effort, together with 12 other universities as well as Meta (formerly Facebook), to achieve the largest egocentric video dataset called Ego4D. It contains 3000+ hours of egocentric video, spanning hundreds of scenarios captured by nearly 1000 unique camera wearers. Ego4D also defines various research tasks for egocentric video understanding, ranging from querying past memory, interpreting current behaviors, and forecasting future tendencies. For example, given a sentence “where and when did I last see my keys?”, the AI system returns the most recent video clip showing where your keys are. Or, the AI system automatically summarizes the video by telling you who is talking and what is his/her main point. Or the AI system predicts where you are walking to and what you are doing in the following minutes or even hours.
For the second aspect, though Ego4D contains baseline solutions to each task, these solutions are far from practical for real-world application. There are two main challenges here. First, current solutions adopt techniques from video understanding tasks for third-person videos (where activities are recorded from a “spectator” view), which are dramatically different from egocentric videos in terms of recording perspective, camera motion, video continuity, etc. As a consequence, representations learned from third-person videos are not optimal to represent egocentric videos. We need to investigate novel feature representations specific to egocentric videos, or explore ways to smartly transfer the knowledge from third-person videos to egocentric videos. Second, egocentric videos pose new challenges for conventional methods due to their characteristics, such as noisy head motion, long videos and fragment actions. We need to address these challenges and improve the performance with novel techniques.
In a nutshell, Ego4D is putting an apron on the robot and knocking on the door of the Metaverse, while at the same time, it is unveiling fresh challenges, which AI researchers are the key. It’s time to hop on board and contribute to this grand effort!
About the Researcher
Bernard Ghanem
Professor, Electrical and Computer Engineering
Affiliations
Education Profile
- Research Scientist, King Abdullah University of Science and Technology (KAUST), 2012
- Senior Research Scientist, Advanced Digital Sciences Center (ADSC) of Illinois in Singapore, 2011
- Postdoctoral Fellow, University of Illinois at Urbana-Champaign (UIUC), 2010
- Ph.D., University of Illinois at Urbana-Champaign (UIUC), 2010
- M.S., University of Illinois at Urbana-Champaign (UIUC), 2008
- B.E., American University of Beirut (AUB), 2005
Research Interests
Professor Ghanem's research interests focus on topics in computer vision, machine learning, and image processing. They include: Modeling dynamic objects in video sequences to improve motion segmentation, video compression, video registration, motion estimation, and activity recognition.Developing efficient optimization and randomization techniques for large-scale computer vision and machine learning problems.Exploring novel means of involving human judgment to develop more effective and perceptually-relevant recognition and compression techniques.Developing frameworks for joint representation and classification by exploiting data sparsity and low-rankness.Selected Publications
- Tianzhu Zhang, Bernard Ghanem, Si Liu, and Narendra Ahuja, ""Robust Visual Tracking via Structured Multi-Task Sparse Learning"", International Journal of Computer Vision (IJCV 2013)
- Tianzhu Zhang, Bernard Ghanem, Si Liu, and Narendra Ahuja, ""Low-Rank Sparse Coding for Image Classification"", International Conference on Computer Vision (ICCV 2013)
- Bernard Ghanem and Narendra Ahuja, ""Dinkelbach NCUT: A Framework for Solving Normalized Cuts Problems with Priors and Convex Constraints"", International Journal of Computer Vision (IJCV 2010)
- Bernard Ghanem and Narendra Ahuja, ""Maximum Margin Distance Learning for Dynamic Texture Recognition"", European Conference on Computer Vision (ECCV 2010)
- Bernard Ghanem and Narendra Ahuja, ""Phase Based Modeling of Dynamic Textures"", International Conference on Computer Vision (ICCV 2007)
Desired Project Deliverables
(i) Effective feature representations of egocentric videos that benefit downstream tasks of egocentric videos, such as episodic memory, future anticipation;
(ii) Novel techniques to transfer/translate between egocentric videos and exocentric videos;
(iii) Improvement to retrieve ‘moments’ from past videos using a category, sentence or an object; (iv) Improvement to identify speaking faces in an egocentric video and summarize the speech.