Deep Learning and Machine Intelligence for Single Cell Genomics
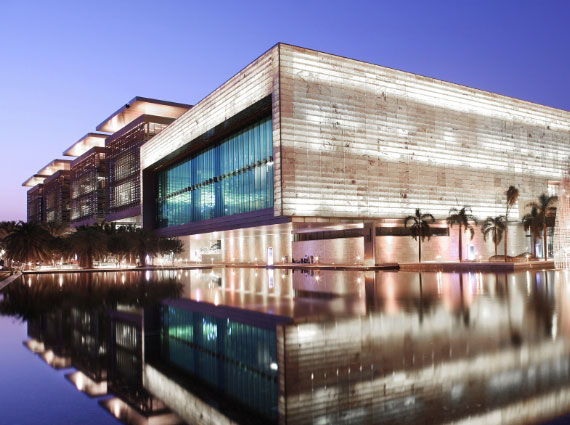
Project Details
Program
BioScience
Field of Study
computer science, bioscience, machine learning, systems biology, artificial intelligence
Division
Biological and Environmental Sciences and Engineering
Faculty Lab Link
Project Description
Single cell biology and genomics in particular are currently transforming the biosciences. Single cell RNA sequencing (scRNAseq), method of the year 2013 (Nature Methods), has now matured and large amounts of scRNAseq are now available. These data, characterizing living systems at an unprecedented level of resolution, hold the promise to set the stage for a fundamental quantitative understanding of living systems with special reference to genomic regulation and collective computation. Yet, there are a number of open problems on how to think about these data and how to pragmatically analyze them.In parallel, we have witnessed a rapid development in machine learning. The rise of computation, such as supercomputers (shaheen@KAUST) and GPU based techniques, in conjunction with data explosion (often referred to as big data), has fuelled the development of new techniques aiming for machine intelligence. In particular, techniques inspired from livings systems, such as deep convolutional networks, currently experience a renaissance. Driving forces include not only data and computation but also the availability of suite of open source platforms (e.g. Theano, Caffe, Torch7, TensorFlow) supporting machine-learning algorithms. These algorithms represent industry standard for processing images, speech, text, and runs on the majority of services and devices provided by Google, Amazon, Facebook, to name a few big players, as well as a numerous startups.We offer internships for several highly motivated bachelor (B.Sc.) or master (M.Sc.) students who will identify (a) appropriate supervised deep learning architectures and training algorithms for scRNAseq data, (b) explore generative adversarial network (GANs) techniques for estimation of high-dimensional data distribution in the single cell gene expression space. This work will be used to develop new techniques and to address open problems in single cell genomics such as pseudo-temporal ordering of single cell data, clustering of data, investigate representations, transfer learning, and unsupervised feature discovery.
About the Researcher
Jesper Tegner
Professor, Bioscience
Affiliations
Education Profile
- Ph.D. Medicine/Medicine Doctor, Karolinska Institutet, 1997
- Advanced Ph.D. courses in pure and computational mathematics (corresponding 2 years full time), Royal Institute for Technology & Stockholm University, 1992-1996
- B.Sc. Medicine (Med Kand, Physician Program), Karolinska Institutet, 1990
- B.Sc. Philosophy, Stockholm University, 1990
- B.Sc. Mathematics, Stockholm University, 1988
Research Interests
Following his PhD (09/1997), he was appointed assistant professor in Computer Science (Dept. of Computer Science and Numerical Analysis, Engineering School, 06/1998). He took a leave of absence, for two postdocs (08/1998-07/2001), Sloan Center for Computational Neuroscience, & Center for Biodynamics, Dept. of Biomedical Engineering, (Boston, US). He was awarded a Swedish Wennergren Foundation Fellowship, 5-year visiting scientist position & faculty position upon return, (first of its kind) & 3-year Alfred P. Sloan Fellowship in Computational Science (US). Upon his return to Sweden (08/2001), awarded a new assistant professor position in Computer Science with special reference to Bioinformatics (Stockholm Center for Bioinformatics), but was awarded a new chaired full professorship in Computational Biology (Dept. of Physics, Engineering School, 02/2002), first of its kind in Sweden. In 10/2009, he was specially recruited to become a strategic chaired full professor in Computational Medicine, appointed a Director for the Computational Medicine Division, at the Dept. of Medicine, Karolinska Institutet & Division of Clinical Epidemiology, Karolinska Hospital. In 06/2014, he was named Faculty at the Science for Life Laboratory (SciLifeLab -A National Center for Molecular Biosciences, Stockholm). Since 08/2016 he is a Professor in Bioscience (BESE) and Professor in Computer Science (CEMSE) at KAUST. He is an ERC co-investigator (2013-) on causal discovery, ranked as outstanding (highest distinction among faculty, ERA 2012) at Karolinska Institutet, winner of the international DREAM competition (2008) on network inference, founder of two BioIT companies, and in 2005 he became the winner of the national award for founding the most promising start-up company of the year. He serves on several editorial boards including being an Associate Editor a- Frontiers in Big Data a- Medicine and Public Health (joint section with Machine Intelligence and Artificial Intelligence), Acting Section Editor on Clinical and Translational Systems Biology in Current Opinion on Systems Biology, Editorial Board of Complex Systems (first in the field, founded 1987 by Stephen Wolfram), Editorial Board of BMC Systems Biology, Senior Editor in Progress in Preventive Medicine, and Editorial Board of Neurology: Neuroinflammation & Neurodegeneration His research targets the circuit architecture and algorithms enabling learning and adaptation in living systems and synthetic machines. Since cells are fundamental building blocks (c.f. atoms in the periodic table) of all living matter, we interrogate their intrinsic circuitry, i.e. networks, by exploiting experimental single cell genomics techniques for temporal multi-molecular profiling, deep imaging, live-cell imaging, and molecular interventions using genomic editing techniques. Such high-dimensional and multi-dimensional data are deciphered by means of advanced bioinformatics, mathematical modeling, and machine learning techniques to uncover the fundamental dynamical equations governing cellular decisions, differentiation, reprogramming, and learning. Theory and algorithms for designing causal discovery machines, are developed by cross-pollinating algorithmic information theory, dynamical systems, inverse modeling, data-driven machine learning techniques, including deep learning architectures. Our applications from this program are threefold; engineered cellular control (reprogramming of stem cells, immune cells, and neurons), software development (data-management, bioinformatics software, causal discovery and machine learning algorithms), and clinical translation (currently Melanoma, Breast Cancer, Multiple Sclerosis, Alzheimer, Frontal Dementia, and Retinal diseases). At the core of our program we posit that such fundamental (causal) dynamical equations drive ""breath of life"" from matter. Since living systems can learn, represent, predict, and in extension understand their local environments, across several orders-of-magnitude of spatial-temporal scales, we believe that the formal deconstruction and reconstruction of such generative mechanisms, evolved over billions of years, will guide the design of algorithmic autonomous learning machines.Selected Publications
- Zenil, Kiani and Tegner. Algorithmic Information Dynamics, Monograph, Cambridge University Press, 2019
- Zenil, Kiani and Tegner. Algorithmic Cognition, Monograph, Springer, 2019
- Zenil, Kiani and Tegner. Graph and Tensor Complexity, Monograph, Springer, 2019
- Zenil et al. A review of graph and network complexity from an algorithmic information perspective, 20, (551), Entropy, 2018
- Kular et al. DNA methylation at HLA as a mediator of the DRB1*15:01 risk haplotype and a novel protective variant in Multiple Sclerosis Nature Communications, 2018
- Zenil et al. A decomposition method for global evaluation of Shannon entropy and local estimation of algorithmic complexity 20, (605) Entropy, 2018
- Kotelnikova et al. Dynamics and heterogeneity of brain damage in multiple sclerosis, PLoS computational biology 13 (10), e1005757, 2017
- H Zenil, NA Kiani, J TegnA©r, Low-algorithmic-complexity entropy-deceiving graphs, Physical Review E 96 (1), 012308, 2017
- Gomez-Cabrero, et al. On the emergence of short advanced courses in Systems Medicine and Biology: recent experiences and recommended guidelines. Cell Systems September, 2017
- David Gomez-Cabrero, and Jesper TegnA©r. Iterative Systems Biology for Medicine a- time for advancing from networks signature to mechanistic equations Current Opinion in Systems Biology May, 2017
- Manuel Zeitelhofera, et al. Functional genomics analysis of vitamin D effects on CD4+ T-cells in vivo in experimental autoimmune encephalomyelitis PNAS February, 2017
- Hill, S. et al. Empirical assessment of causal network learning through a community-based effort Nature Methods, 2016.
- RodrAguez-Cortez, et al. Monozygotic twins discordant for common variable immunodeficiency reveal impaired DNA demethylation during naA¯ve-to-memory B-cell transition Nature Communications 6/17, 2015.
- Yang, et al. VEGF-B promotes cancer metastasis through a VEGF-Aa-independent mechanism and serves as a marker of poor prognosis for cancer patients PNAS 5/19, 2015.
- Lindholm, et al. An integrative analysis reveals coordinated reprogramming of the epigenome in human skeletal muscle after training Epigenetics.12/2, 1557-69. 2014
- Gomez-Cabrero et al. Data integration in the era of omics: current and future challenges. BMC Systems Biology, vol 8 (Supp 2):I1), 2014
- Marabita, et al. An evaluation of analysis pipelines for DNA methylation profiling using the Illumina HumanMethylation450 BeadChip platform Volume 8, Issue 3 Epigenetics March, 8(3), 333a-46., 2013
- Teschendorff et al. S A beta-mixture quantile normalization method for correcting probe design bias in Illumina Infinum 450k DNA methylation data Bioinformatics. Jan 15;29(2):189-96, 2013
- Ravasi et al. An atlas of combinatorial transcriptional regulation in mouse and man, Cell. Mar 5;140(5):744-52. 2010
- Suzuki et al. A complex transcriptional network controls growth arrest and differentiation in a human myeloid leukemia cell line, Nature Genetics May;41(5):553-62. Epub 2009 Apr 19, 2009
- Pena, J., Nilsson, R, BjA¶rkegren, J. and TegnA©r, J. An Algorithm for Reading Dependencies from the Minimal Undirected Independence Map of a Graphoid that Satisfies Weak Transitivity. Journal of Machine Learning Research 2009.
- Fredrik Edin, et al. Mechanism for Top-down Control of Working Memory Capacity. PNAS Apr 21;106(16):6802-7. Epub Apr 1. 2009
- PeA±a, J. M., Nilsson, R., BjA¶rkegren, J. and TegnA©r, J. Towards scalable and Data Efficient Learning of Markov Boundaries, International Journal of Approximate Reasoning, Volume 45, Issue 2, Pages 211-232 2007
- TegnA©r, J. and BjA¶rkegren, J. Perturbations to uncover gene networks. Trends in Genetics, Jan;23(1):34-41, 2007
- Nilsson, R., PeA±a, J. M., BjA¶rkegren J., and J. TegnA©r, Consistent feature selection for pattern recognition in polynomial time, Journal of Machine Learning Research, 8(March):589-612, 2007
- Carninci, T. et al. The transcriptional landscape of the mammalian genome, Science. Sep 2;309 (5740):1559-63. 2005
- TegnA©r, J., et al. Reverse engineering gene networks -- integrating genetic perturbations with dynamical modeling. PNAS. 100,5944-5949, 2003.
Desired Project Deliverables
Individual projects will be tailored and narrowly designed from the above palette according to interest of the student, technical proficiency, and level of study. The project is suitable for candidates fascinated of living systems, interested in cutting edge bioscience, and artificial intelligence for science and not for discovering cats in YouTube. We expect you (a) to bring enthusiasm, creativity, and hard work, (b) give lab seminars on your work, and (c) produce a final written report.In returnthis facilitates your critical thinking, presentations skills, and scientific writing.Yourresearch, in collaboration and with support of team members, may lead to scientific publications. We publish avidly in both bioscience and computational sciences, not for the fame but rather as steps aiming to and motivated both by our quest of asking fundamental questions of relevance to human nature and discovery of transformative intelligent technologies inspired from nature. You will also get a good hands-on perspective at the frontier of bioscience and machine intelligence in an interdisciplinary research group and environment.